DOWNFALL OF CORPORATIONS
- Companies can go out of business for poor management, wrong decisions, changing times or if they get caught in dishonest and illegal activities which are termed as corporate fraud.
- The fraudulent activities include falsified accounting, forged documents, share rigging, misrepresenting services or products.
The Enron (US) scandal from 2001 was a result of series of dubious accounting practices
Lehman brothers bankruptcy in 2008 can be attributed to faulty decision and downturn of US real estate market
Satyam Computer Services (India) fraud in 2009 was from falsification of revenue, margin and cash balances
Theranos scandal (US) in 2018 for audacious lies on product
REGULATORY BODIES
- When a company goes out of business the impact is disastrous to economy. Larger the size of the company, bigger is the blow.
- Not only the stockholders experience loss of wealth, but also the employees find themselves in uncertain circumstances with possible loss of retirement benefits.
- To protect the interests of investors and prevent fraudulent practices, governments around the world formed regulatory bodies.
FINANCIAL REGULATORY AUTHORITIES BY COUNTRY
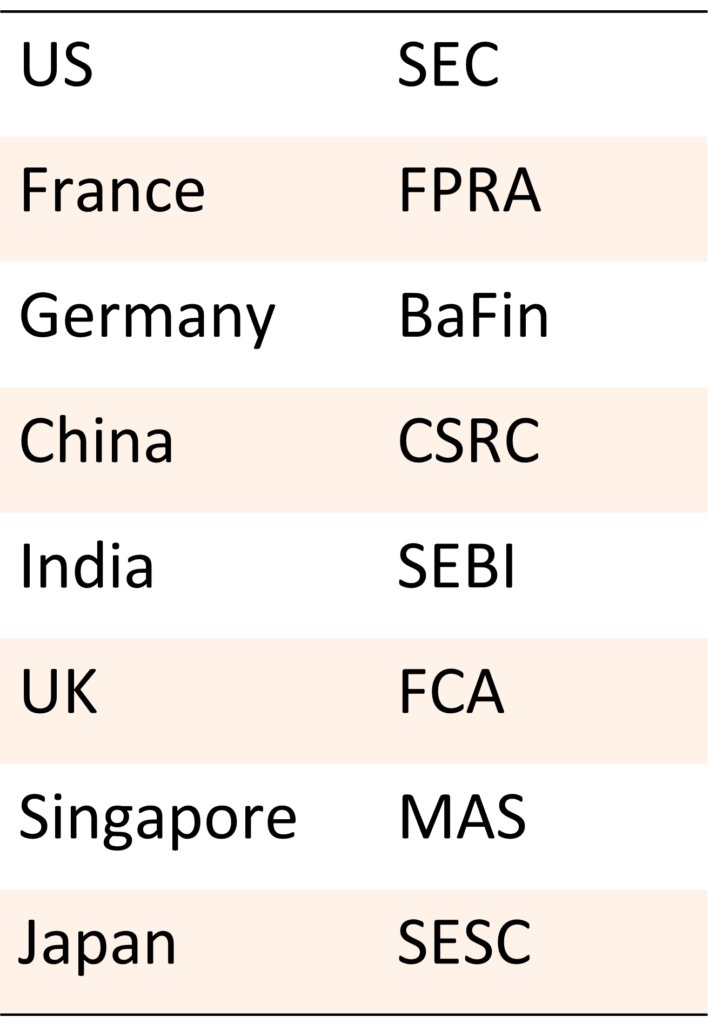
CASE STUDY
- An online platform provides a structured database with information of registered companies on finance, key personnel, business activities, litigation, news, and board members.
- The beneficiaries of this data include NBFCs, private banks, mutual funds, and private equity funds.
- With this rich repository of information, the company created an expert score mimicking a credit rating to keep vigil on the companies to avoid financial disaster. Any shock in the daily score would raise a flag if something is amiss.
TO DO
Calibrate expert score with ML methodologies
Automate day-to-day score generation
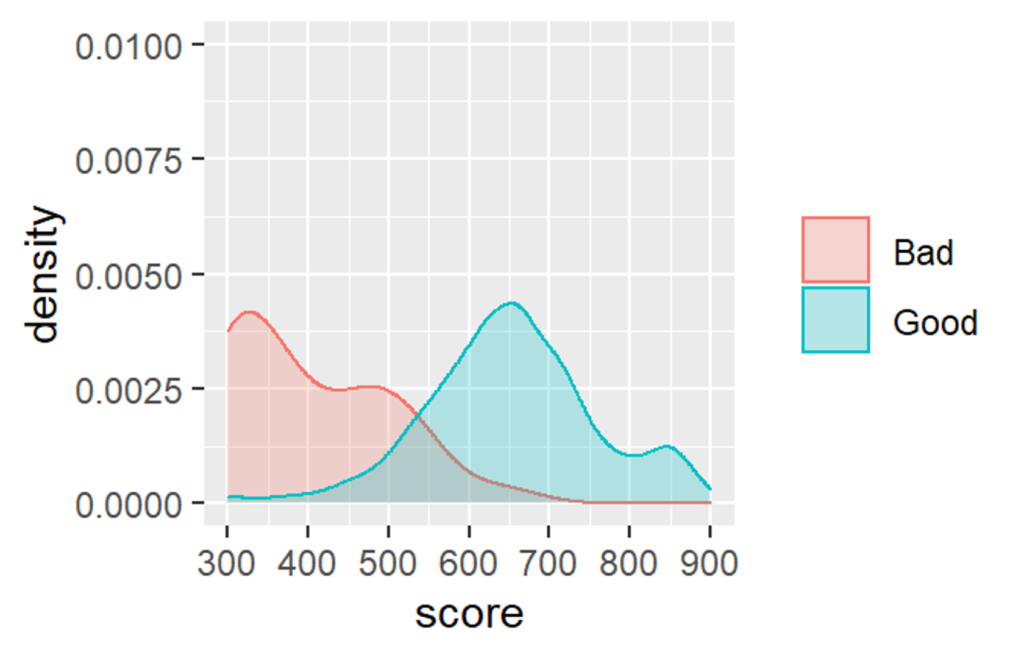
MODELLING METHODOLOGY
- Company default was defined based on various criteria, like company rating, willful default, company audit report order, and NCLT cases.
- The model was trained on historical data spanning 4 years.
- ML models included Random Forest and Gradient Boosting. Shows good separation of defaulters.
- Separate models for listed and unlisted companies to address the issue of lack of data for the unlisted.
- Decision overlay on final scoring for fine tuning.
OBJECTIVES OF CREDIT MONITORING
- The objective of the Credit Monitoring and Default Prediction System is to provide an advanced and automated tool for assessing the credit risk of Listed and Unlisted Companies in a timely manner, empowering lenders and investors to make informed decisions. The system utilizes predictive technology to identify companies at risk of default well in advance, offering a 90 to 360 days response time for effective remedial measures.
- Machine Learning Analysis: Developed machine learning modals to identify patterns, categorizing companies on a risk scale from very high to very low.
- Default Prediction Accuracy: It achieves over 90% accuracy in predicting potential defaults, instilling confidence in decision-making.
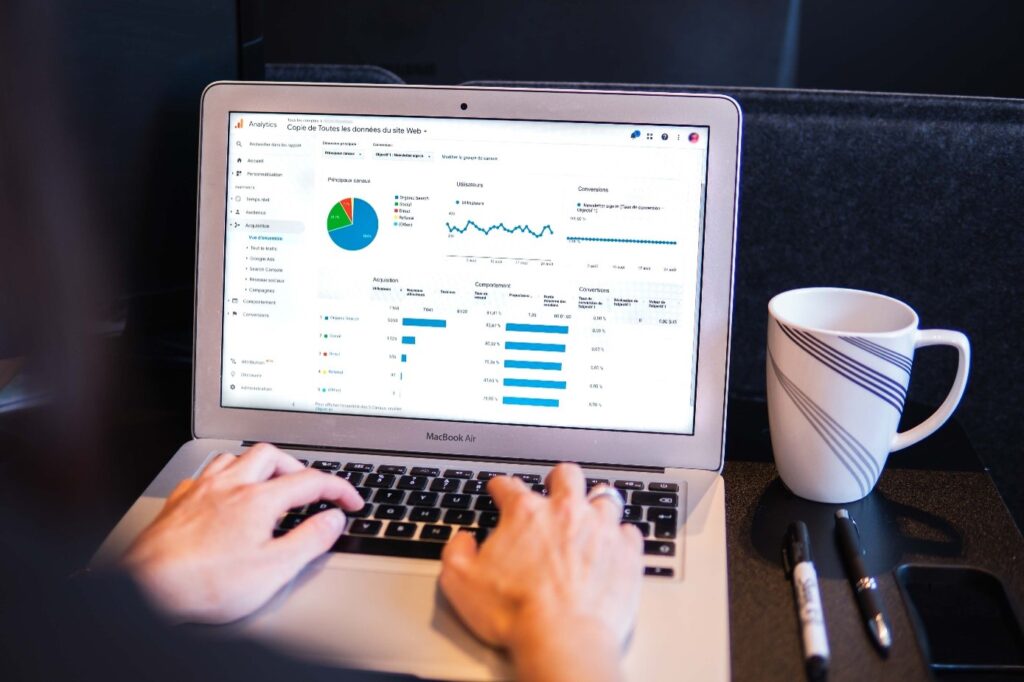
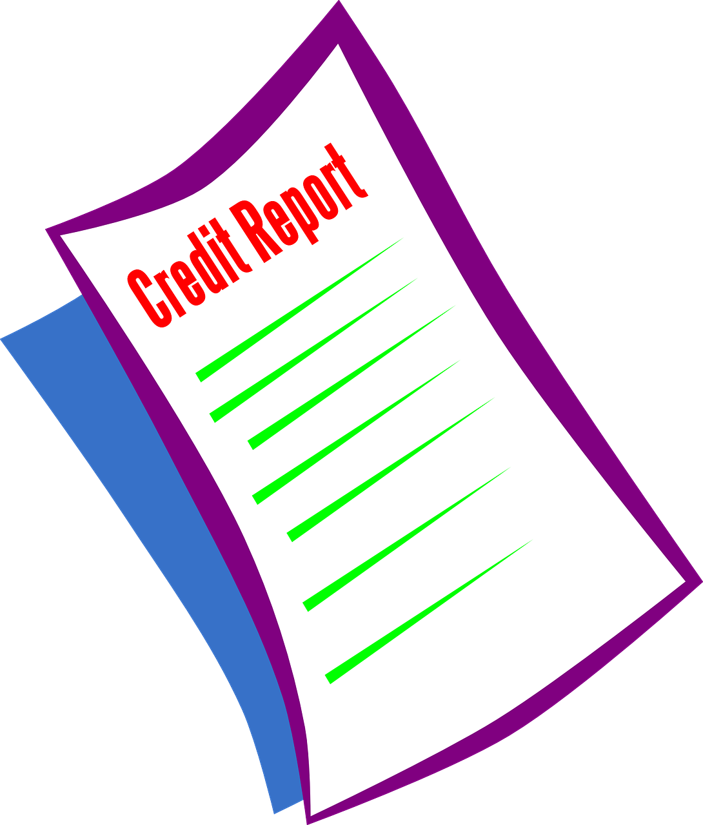
OBJECTIVES OF CREDIT MONITORING
- Event Pattern Recognition: Identifies event patterns, converting them into a quantifiable Risk Score for nuanced risk assessment.
- In-Depth Analysis: Provides detailed analyses of risk-triggering data, offering insights into specific risk factors.
- Auto-Alert Mechanism: Automated alerts ensure timely updates on portfolio risk changes, enabling proactive risk management.
- Audience: Designed for lenders, investors, and stakeholders seeking enhanced credit decision support.
BENEFITS
Process automation
All changes in data are readily reflected in the score appearing on the dashboard.
Continuous monitoring
Any abnormal activities of a company are instantaneously detected from trend graphs daily scores.